Implementing Generative AI in Banking: Challenges & Path to Success
- Balaji Sampathkumar
- Sep 29, 2024
- 4 min read
Updated: Feb 28
AI is changing banking. Fast.
Fraud detection, customer service, risk assessment—AI is everywhere.
But here's the hard truth: Most AI projects fail. Why? Poor planning. Bad execution. Resistance to change.
Banks that rush in without a roadmap? They waste millions. Banks that strategize, integrate, and govern AI effectively? They lead. This is how to get it right.
The Five Biggest AI Challenges in Banking
AI isn't just a technology shift—it's an operational transformation.
Challenge 1: Data Privacy & Security Risks
Banks run on data. AI thrives on data. But data security is non-negotiable.
Massive data exposure → AI needs vast datasets, but mishandling risks leaks.
Regulatory minefield → GDPR, CCPA, and banking-specific rules demand airtight compliance.
Cyber threats → AI-driven systems are lucrative targets for hackers.
Banks must:
Encrypt sensitive data.
Anonymize AI training datasets.
Implement zero-trust cybersecurity frameworks.
If AI compromises security, it fails.
Challenge 2: AI Ethics & Bias in Decision-Making
AI can be brilliant. It can also be biased.
Unfair lending decisions → AI can unknowingly favour certain demographics.
Opaque decision-making → Many AI models act as "black boxes."
Customer trust erosion → Bias leads to backlash.
Banks must:
Audit AI models for fairness.
Use explainable AI—transparency builds trust.
Regulate AI outputs—human oversight is critical.
Without ethics, AI destroys reputations.
Challenge 3: Legacy System Integration Nightmare
Most banks still rely on decades-old infrastructure.
Outdated tech stacks → Can't support AI workloads.
Data silos → Fragmented customer data hinders AI-driven insights.
Complex integrations → AI adoption requires serious IT upgrades.
Banks must:
Invest in cloud-first architecture.
Break down data silos for seamless AI access.
Deploy AI incrementally—not all at once.
AI must work with legacy systems, not fight against them.
Challenge 4: Employee Resistance & Skill Gaps
AI won't replace bankers—but many fear it will.
Lack of AI fluency → Employees resist what they don't understand.
Fear of job loss → AI's automation raises job security concerns.
Slow adoption → Without buy-in, AI projects stall.
Banks must:
Train employees on AI's role as an enabler, not a replacement.
Foster a culture of upskilling—AI literacy is now essential.
Deploy AI in ways that enhance employee roles, not eliminate them.
AI adoption isn't just about technology—it's also about people.
Challenge 5: Regulatory & Compliance Barriers
Banking is one of the most regulated industries. AI doesn't change that.
AI-driven decisions need regulatory clarity.
Automated processes must meet compliance standards.
Regulators demand explainability & control.
Banks must:
Align AI initiatives with existing regulatory frameworks.
Develop compliance-first AI models.
Partner with regulators early—collaboration beats conflict.
If AI can't meet compliance, it's a liability, not an asset.
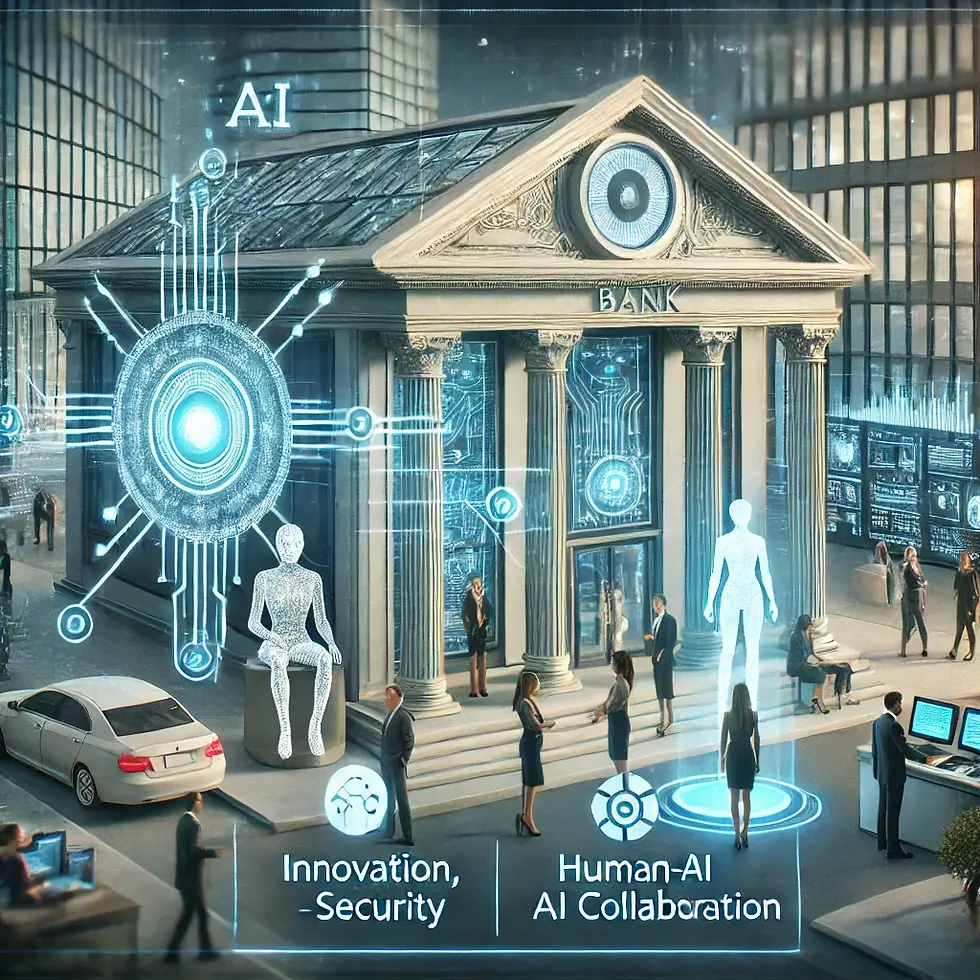
Path to Success: How to Implement AI the Right Way
Avoiding failure isn't enough. Banks must execute AI flawlessly. This is how to do it right.
Step 1: Phased AI Implementation
Rushing AI creates chaos. A step-by-step approach ensures success.
Phase 1: AI Readiness Assessment → Audit data, systems, and workforce skills.
Phase 2: Pilot Projects → Start with one high-impact area (fraud, chatbots, risk models).
Phase 3: Scale Gradually → Expand AI based on pilot success.
Phase 4: Continuous Optimization → AI improves over time, not overnight.
Banks that implement AI in waves see the highest ROI.
Step 2: Choosing the Right AI Tools & Partners
Not all AI platforms are built for banking.
Banks need:
Scalability → AI must handle millions of transactions.
Security & Compliance → Built-in regulatory controls.
Interoperability → Seamless integration with core banking systems.
Best AI investment? Strategic partnerships.
AI vendors with banking expertise.
Tech providers with proven models.
Cloud-based AI for faster deployment.
Choose AI solutions that fit your bank's ecosystem.
Step 3: AI Governance & Ethics Frameworks
AI needs rules. Without governance, banks lose control.
Key governance areas:
Accountability → Who owns AI outcomes?
Bias & Fairness Audits → AI decisions must be ethical and unbiased.
Explainability Requirements → No "black box" decisions.
Best practice? Establish AI Ethics Committees—oversight ensures responsible AI.
Step 4: Measuring AI's ROI (Key Metrics)
AI should make banking better. But how do you measure success?
KPIs that matter:
Customer Satisfaction → Faster response times, higher engagement.
Operational Efficiency → Cost savings, reduced manual errors.
Fraud Prevention → AI-driven fraud detection rates.
Regulatory Compliance → AI-driven compliance accuracy.
If AI isn't improving these metrics, it's failing.
Step 5: Future-Proofing AI in Banking
AI isn't static. It evolves.
Banks must:
Stay ahead of AI advancements.
Continuously improve AI models.
Scale AI with business growth.
AI success isn't about the first year but long-term adaptability.
The AI-Powered Bank: What Success Looks Like
Imagine a bank where AI seamlessly enhances every operation.
Customers get personalized service instantly.
Fraud detection operates in real-time, preventing threats.
Regulatory compliance is automated and risk-free.
Bankers focus on strategic work—AI handles the rest.
This isn't the future—it's happening now.
Final Thoughts: AI in Banking Isn't Optional. It's Inevitable.
🔹 Banks that strategize AI adoption win.
🔹 Banks that ignore AI fall behind.
The choice is simple:
✅ Lead the AI revolution.
❌ Get left behind.
Citations:
[2] https://www.finextra.com/blogposting/24293/why-implementing-generative-ai-is-challenging-for-banks
[4] https://www.linkedin.com/pulse/impact-ai-employment-skill-demands-banking-sector-workassist-in-0nxec
Comments